Frontier Research Overview
How can brain-like mechanisms be developed and realized on artificial systems so they can perform multiple complex functions as biological living systems?
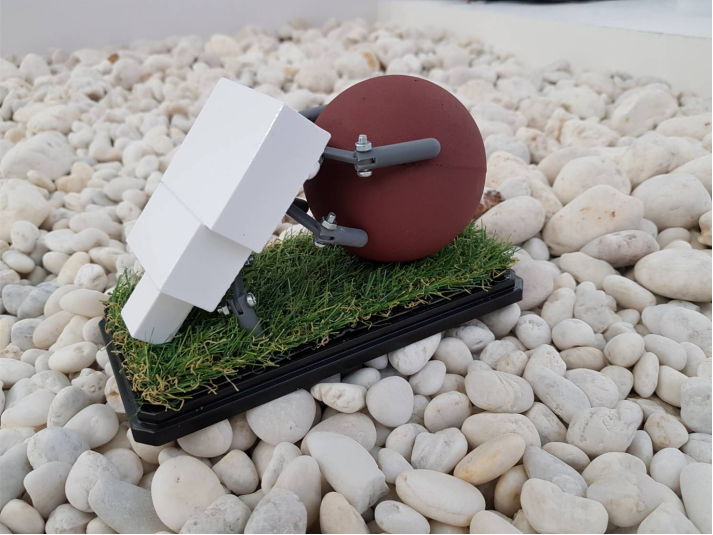
To address this fundamental question, we employ a bio-inspired approach to develop brain-like mechanisms for adaptive motor control and autonomous learning of embodied multi-sensorimotor robotic systems. The developed mechanisms (BRAIN technology) are adaptive and flexible, which can be transferred to application areas like human-machine interaction, brain-machine interface, and rehabilitation.
Further information: click!
Research Highlights
2023
Proactive Body Joint Adaptation for Energy-Efficient Locomotion of Bio-Inspired Multi-Segmented Robots
Abstract: Typically, control strategies for legged robots have been developed to adapt their leg movements to deal with complex terrain. When the legs are extended in search of ground contact to support the robot body, this can result in the center of gravity (CoG) being raised higher from the ground and can lead to unstable locomotion if it deviates from the support polygon. An alternative approach is body adaptation, inspired by millipede/centipede locomotion behavior, which can result in low ground clearance and stable locomotion. In this study, we propose novel proactive neural control with online unsupervised learning, allowing multi-segmented, legged robots to proactively adapt their body to follow the surface contour and maintain efficient ground contact. Our approach requires neither kinematics nor environmental models. It relies solely on proprioceptive sensory feedback and short-term memory, enabling the robots to deal with complex 3D terrains. In comparison to traditional reflex-based control, our approach results in smoother and more energy-efficient robot locomotion on terrains with concave and convex curves or slopes of varying degrees in both simulation and real-world implementation.
Reference: Homchanthanakul, J.; Manoonpong, P. (2023) Proactive Body Joint Adaptation for Energy-Efficient Locomotion of Bio-Inspired Multi-Segmented Robots, IEEE Robotics and Automation Letters (RAL), doi: 10.1109/LRA.2023.3234773 (see more)
Self-Organized Stick Insect-Like Locomotion under Decentralized Adaptive Neural Control: From Biological Investigation to Robot Simulation
Abstract: Living animals and legged robots share similar challenges for movement control. In particular, the investigation of neural control mechanisms for the self-organized locomotion of insects and hexapod robots can be informative for other fields. The Annam stick insect Medauroidea extradentata is used as a template to develop a biorobotic model to infer walking self-organization with strongly heterogeneous leg lengths. Body dimensions and data on the walking dynamics of the actual stick insect are used for the development of a neural control mechanism, generating self-organized gait patterns that correspond to the real insect observations. The combination of both investigations not only proposes solutions for distributed neural locomotion control but also enables insights into the neural equipment of the biological template. Decentralized neural central pattern generation is utilized with phase modulation based on foot contact feedback to generate adaptive periodic base patterns and a radial basis function premotor network in each leg based on the target trajectories of actual stick insect legs during walking for complex intralimb coordination and self-organized interlimb coordination control. Furthermore, based on both study objects, a robot with heterogeneous leg lengths is constructed to preliminary validate the findings from the simulations and real insect observations.
Reference: A. Larsen, T. B ̈uscher, T. Chuthong, T. Pairam, H. Bethge, S. Gorb, P. Manoonpong, Advanced
Theory and Simulations 2023, 6. (see more)
Nature Inspired Machine Intelligence from Animals to Robots
Abstract: In nature, living creatures show versatile locomotion on various terrains. They can also perform impressive object manipulation/transportation using their legs. Inspired by their morphologies and control strategies, we have developed various bio-inspired robots and modular neural mechanisms for controlling robot locomotion and object manipulation/transportation behaviors. In this video, we demonstrate the recent results of our five bio-inspired robots and their special abilities in our robot zoo setup. Inchworm-inspired robots with two electromagnetic feet (Freelander-02 and AVIS) can adaptively crawl and balance on horizontal and vertical metal pipes. Their crawling behavior and locomotion transition from horizontal to vertical terrain follow inchworm locomotion strategies. With special design, the Freelander-02 robot can adapt its posture to crawl underneath an obstacle, while the AVIS robot can step over a flange. A millipede-inspired robot with multiple body segments (Freelander-08) can proactively adapt its body joints to efficiently navigate on bump terrain. A dung beetle-inspired robot (ALPHA) can transport an object by grasping the object with its hind legs and at the same time walk backward with the remaining legs like dung beetles. Finally, an insect-inspired robot (MORF), which is a hexapod robot platform, demonstrates typical insect-like gaits (e.g., slow wave and fast tripod gaits). In a nutshell, we believe that this bio-inspired robot zoo demonstrates how the diverse and fascinating abilities of living creatures can serve as inspiration and principles for developing robotics technology capable of achieving multiple robotic functions and solving complex motor control problems in systems with many degrees of freedom.
Reference: Chuthong, T.; Ausrivong, W.; Leung, B.; Homchanthanakul, J.; Mingchinda, N.; Manoonpong, P. (2023) Nature Inspired Machine Intelligence from Animals to Robots, Stand-alone video, 2023 IEEE International Conference on Robotics and Automation (ICRA)
Insect Tarsus-Inspired Compliant Robotic Gripper With Soft Adhesive Pads for Versatile and Stable Object Grasping
Abstract:
Grasping multiple object types (versatile object grasping) with a single gripper is always a challenging task in robotic manipulation. Different types of grippers, including rigid and soft, have been developed to try to achieve the task. However, each gripper type is still restricted to specific object types. In nature, many insects can be observed to use only one tarsus mechanism to cope with several tasks. They have a very high grasping capability with objects and can adhere to a variety of surface types. Inspired by insect tarsus, this paper proposes a novel underactuated, single cable-driven, compliant gripper design. The structure of the gripper is based on the hornet tarsus morphology with a proportional scale. An additional pulley-like structure is introduced to increase the generated grasping torque. To maintain the ability to automatically rebound back to the original position, a torsion spring is implemented at each joint. In order to stably grasp and hold objects, soft adhesive pads with an asymmetric sawtooth-like surface structure are attached at the tarsus segments. The performance of this insect tarsus-inspired gripper with the soft pads is evaluated by grasping 35 different objects of various sizes, shapes, and weights for comparison with industrial soft and rigid grippers. The proposed gripper shows a 100% success rate in grasping all objects, while the soft and rigid gripper success rates are 81.90% and 91.43% on average, respectively. We finally demonstrate the use of our gripper installed on a robot arm for pick-and-place and pouring tasks.
For more details, see Phodapol et al., RAL/IROS, 2023.
Integrated Modular Neural Control for Versatile Locomotion and Object Transportation of a Dung Beetle-Like Robot
Abstract:
Dung beetles can effectively transport dung pallets of various sizes in any direction across uneven terrain. While this impressive ability can inspire new locomotion and object transportation solutions in multilegged (insect-like) robots, to date, most existing robots use their legs primarily to perform locomotion. Only a few robots can use their legs to achieve both locomotion and object transportation, although they are limited to specific object types/sizes (10%–65% of leg length) on flat terrain. Accordingly, we proposed a novel integrated neural control approach that, like dung beetles, pushes state-of-the-art insect-like robots beyond their current limits toward versatile locomotion and object transportation with different object types/sizes and terrains (flat and uneven). The control method is synthesized based on modular neural mechanisms, integrating central pattern generator (CPG)-based control, adaptive local leg control, descending modulation control, and object manipulation control. We also introduced an object transportation strategy combining walking and periodic hind leg lifting for soft object transportation. We validated our method on a dung beetle-like robot. Our results show that the robot can perform versatile locomotion and use its legs to transport hard and soft objects of various sizes (60%–70% of leg length) and weights (approximately 3%–115% of robot weight) on flat and uneven terrains. The study also suggests possible neural control mechanisms underlying the dung beetle Scarabaeus galenus’ versatile locomotion and small dung pallet transportation.
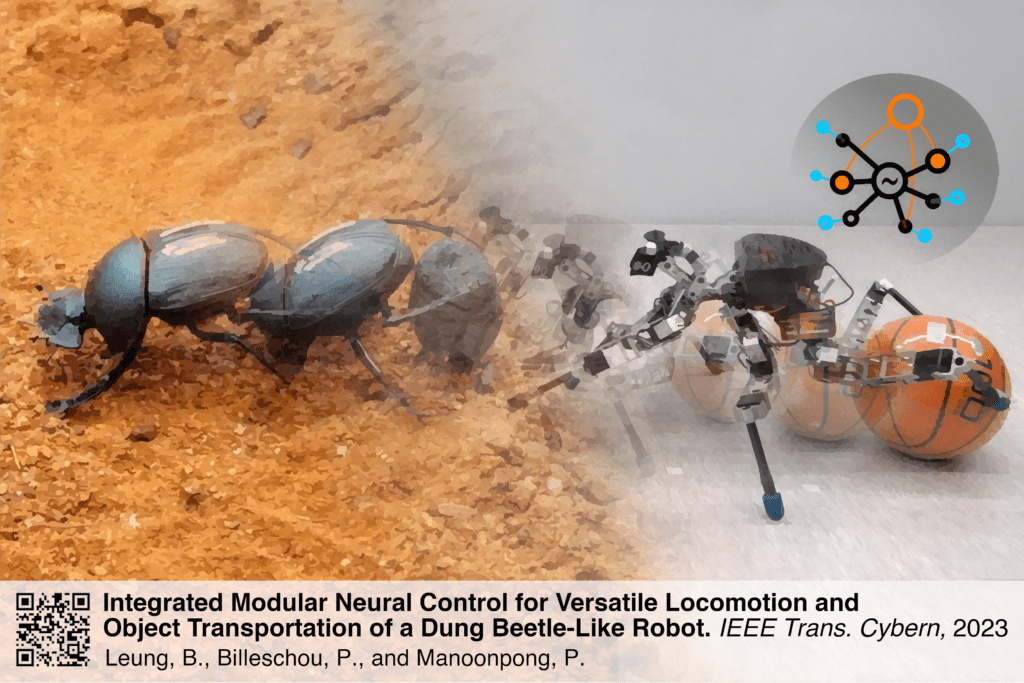
Reference: B. Leung, P. Billeschou and P. Manoonpong, IEEE Transactions on Cybernetics. (see more)
2022
Nature-Inspired Machine Intelligence: From Animals to Robots
Credit : สํานักข่าวไทย TNAMCOT : Youtube link
GRAB: GRAdient-Based Shape-Adaptive Locomotion Control
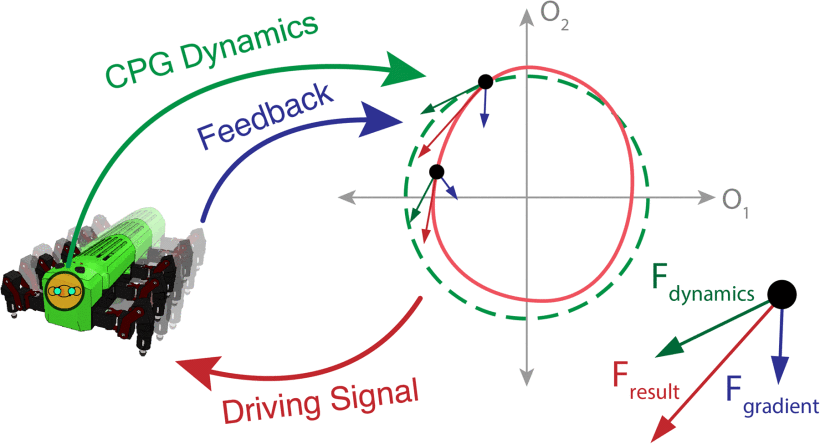
Adaptive systems enable legged robots to cope with a wide range of environmental settings and unforeseen events. Existing reactive methods adapt either the walking frequency or the amplitude to only simple perturbations. This letter proposes an adaptive mechanism for central pattern generator (CPG)-based locomotion control that online-reacts to both internal and external soft constraints by adapting both the frequency and amplitude of driving signals. Our approach, namely GRAdient-Based shape adaptive control (GRAB), utilises real-time sensory signals for adapting the dynamics of the CPG. GRAB reacts to locomotion soft constraints given in a loss function. It can quickly adapt CPG’s dynamics variables to reduce such a loss, with a gradient-descent-like update step. The update perturbs the shape of the driving signal, which implicitly changes both frequency and amplitude of the robot locomotion pattern. We test the GRAB mechanism on a hexapod robot and its simulation, where we demonstrate its several benefits over a state-of-the-art adaptive control baseline. First, we show that it can be used for reducing the tracking error by simultaneously changing the walking amplitude and frequency. Also, GRAB can be used for limiting the maximum torque/current, preventing motor damage from unexpected perturbations. Finally, we demonstrate how GRAB can be utilised to naturally adjust the robot’s walking speed while taking into account multiple constraints, including target walking speed, external weight perturbations, and the robot’s physical limit. A video of this research.
For more details, see Phodapol et al., ICRA/RAL, 2022.
Continuous Online Adaptation of Bioinspired Adaptive Neuroendocrine Control of Autonomous Walking Robots
We developed an advanced control method with short-term memory for complex locomotion and lifelong adaptation of autonomous walking robots. The control method is inspired by a locomotion control strategy used by walking animals like cats, in which they use their short-term visual memory to detect an obstacle and take proactive steps to avoid colliding it. Using this control method allows a hexapod robot to traverse complex terrains and perform proactive leg movements to swing over an obstacle before hitting it. This robot control technology will increase the robot’s agility for real-world applications. A video of this research.
For more details, see Homchanthanakul et al., IEEE TNNLS, 2021.
Gait Adaptation of a Dung Beetle Rolling a Ball up a Slope
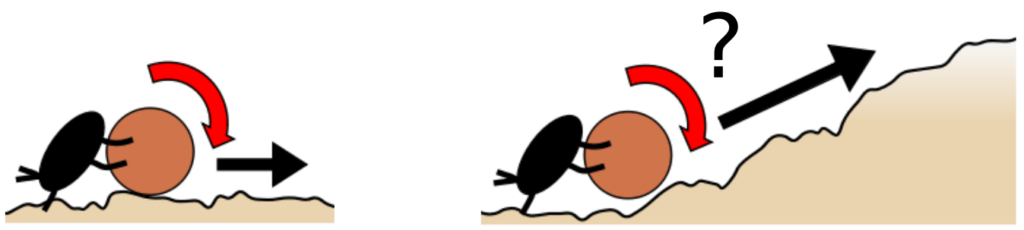

A previous study describes the gait pattern of the ball rolling behavior on flat terrain, but little is known how the dung beetles adapt their movement to roll a ball up a slope. Thus, in this work, we perform a visual investigation of dung beetles’ ball rolling behavior on 0 and 20-degree slopes and perform statistical analysis on the gait patterns to identify how dung beetles adapt their movement to roll a ball up a slope. We found that the dung beetle’s front legs and hind legs tend to stay in contact with the ground and dung ball more often in the 20-degree slope than in the 0-degree slope condition. A video of this research talk.
2020
Rules for the Leg Coordination of Dung Beetle Ball Rolling Behaviour
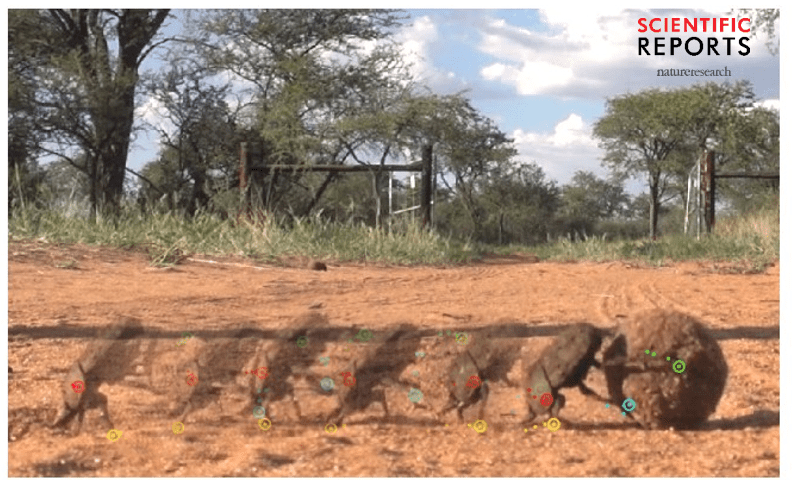
The dung beetle is particularly strong insect that can transport a large and heavy dung ball across the savanna. Through behavioral experiments and statistical analysis, we successfully reveal the secret rules that dung beetles use to transport a ball. This study can be used as a basis to push robot technology beyond the state of the art; thereby creating a next-generation robot with agility and versatility. “Imagine if we could build a similarly effective robot that could walk and transport an object ten times its own weight, like the beetle”.
For more details, see Leung et al., Scientific Reports, 2020.
A video link of the dung beetle experiments
Dynamical State Forcing on Central Pattern Generators
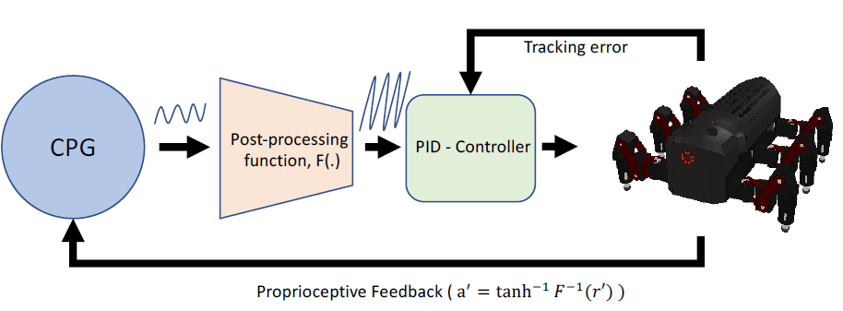
Many CPG-based locomotion models have a problem known as the tracking error problem, where the mismatch between the CPG driving signal and the state of the robot can cause undesirable behaviors for legged robots. Towards alleviating this problem, we introduce a mechanism that modulates the CPG signal using the robot’s interoceptive information. The key concept is to generate a driving signal that is easier for the robot to follow, yet can drive the locomotion of the robot. This can be done by nudging the CPG signal in the direction of lower tracking error, which can be analytically calculated. Unlike other reactive CPG, the proposed method does not rely on any parametric learning ability to adjust the shape of the signal, making it a unique option for a biological adaptive motor control.
For more details, see Chuthong et al., ICONIP 2020. Lecture Notes in Computer Science, 2020.
Video link:
2018
Modular Neural Control for Bio-Inspired Walking and Ball Rolling of a Dung Beetle-Like Robot
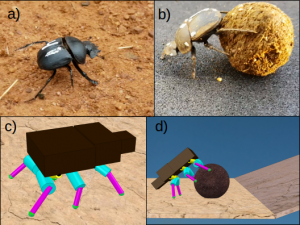
Dung beetles can perform impressive multiple motor behaviors using their legs. The behaviors include walking and rolling a large dung ball on different terrains, e.g., level ground and different slopes. To achieve such complex behaviors for legged robots, we propose here a modular neural controller for dung beetle-like locomotion and object transportation behaviors of a dung beetle-like robot. The modular controller consists of several modules based on three generic neural modules. The main modules include 1) a neural oscillator network module (as a central pattern generator (CPG)), 2) a neural CPG postprocessing module (PCPG), 3) a velocity regulating network module (VRN). The CPG generates basic rhythmic patterns. The patterns are first shaped by the PCPG and their amplitudes as well as phases are later modified by the VRN to obtain proper motor patterns for locomotion and object transportation. Combining all these neural modules, we can achieve different motor patterns for four different actions which are forward walking, backward walking, levelground ball rolling, and sloped-ground ball rolling. All these actions can be activated by four input neurons. The experimental results show that the simulated dung beetle-like robot can robustly perform the actions. The average forward speed is 0.058 cm/s and the robot is able to roll a large ball (about 3 times of its body height and 2 times of its weight) up different slope angles up to 25 degrees.
For more details, see Leung et al., ALIFE., 2018.